Tools for MLN evaluation¶
PRACMLNs comes with a set of tools for convenient evaluation of an MLN or learning/inference algorithms.
Confusion Matrices¶
-
class
pracmln.utils.eval.
ConfusionMatrix
¶ Represents a confusion matrix and provides some convenience methods for computing statistics like precision, recall, F1 score or methods for creating LaTex output.
-
addClassificationResult
(prediction, groundTruth, inc=1)¶ Add a new classification result to the confusion matrix.
gndTruth: the correct label of an example
prediction: the predicted class label of an example
inc: the increment (default: 1)
-
combine
(matrix)¶ Combines another confusion matrix with this one.
-
countClassifications
(classname)¶ Returns the true positive, true negative, false positive, false negative classification counts (in this order).
-
getLatexTable
()¶ Returns LaTex code for the confusion matrix.
-
getMatrixEntry
(pred, clazz)¶ Returns the matrix entry for the prediction pred and ground truth clazz.
-
getMetrics
(classname)¶ Returns the classifier evaluation metrices in the following order: Accuracy, Precision, Recall, F1-Score.
-
getTotalAccuracy
()¶ Returns the fraction of correct predictions and total predictions.
-
iteritems
()¶ Iterates over triples of the form (prediction, class, count) of this confusion matrix.
-
printPrecisions
()¶ Prints to the standard out a table of the class-specific error measures accurracy, precision, recall, F score.
-
printTable
()¶ Prints the confusion matrix nicely formatted onto the standard out.
-
toFile
(filename)¶ Pickles the confusion matrix to a file with the given name.
-
toPDF
(filename)¶ Creates a PDF file of this matrix. Requires ‘pdflatex’ and ‘pdfcrop’ installed.
-
Examples¶
>>> cm = ConfusionMatrix()
>>> for _ in range(10):
... cm.addClassificationResult("AAA","A")
>>> cm.addClassificationResult("AAA","AAA")
>>> cm.addClassificationResult("AAA","AAA")
>>> cm.addClassificationResult("AAA","AAA")
>>> cm.addClassificationResult("AAA","AAA")
>>> cm.addClassificationResult("AAA","B")
>>> cm.addClassificationResult("AAA","B")
>>> cm.addClassificationResult("AAA","C")
>>> cm.addClassificationResult("B","AAA")
>>> cm.addClassificationResult("B","AAA")
>>> cm.addClassificationResult("B","C")
>>> cm.addClassificationResult("B","B")
>>> cm.printTable()
-------------------------------
| P\C | A | AAA | B | C |
|-----+-----+-----+-----+-----|
| A | 0 | 0 | 0 | 0 |
|-----+-----+-----+-----+-----|
| AAA | 10 | 4 | 2 | 1 |
|-----+-----+-----+-----+-----|
| B | 0 | 2 | 1 | 1 |
|-----+-----+-----+-----+-----|
| C | 0 | 0 | 0 | 0 |
-------------------------------
>>> cm.printPrecisions()
A: - Acc=0.52, Pre=0.00, Rec=0.00 F1=0.00
AAA: - Acc=0.29, Pre=0.24, Rec=0.67 F1=0.35
B: - Acc=0.76, Pre=0.25, Rec=0.33 F1=0.29
C: - Acc=0.90, Pre=0.00, Rec=0.00 F1=0.00
>>> cm.getLatexTable()
\footnotesize
\begin{tabular}{|l|l|l|l|l|}
\hline
Prediction/Ground Truth & \begin{turn}{90}A\end{turn} & \begin{turn}{90}AAA\end{turn} & \begin{turn}{90}B\end{turn} & \begin{turn}{90}C\end{turn}\\ \hline
A & \cellcolor{cfmcolor!0}\textbf{0} & \cellcolor{cfmcolor!0}0 & \cellcolor{cfmcolor!0}0 & \cellcolor{cfmcolor!0}0\\ \hline
AAA & \cellcolor{cfmcolor!53}10 & \cellcolor{cfmcolor!21}\textbf{4} & \cellcolor{cfmcolor!11}2 & \cellcolor{cfmcolor!5}1\\ \hline
B & \cellcolor{cfmcolor!0}0 & \cellcolor{cfmcolor!33}2 & \cellcolor{cfmcolor!17}\textbf{1} & \cellcolor{cfmcolor!17}1\\ \hline
C & \cellcolor{cfmcolor!0}0 & \cellcolor{cfmcolor!0}0 & \cellcolor{cfmcolor!0}0 & \cellcolor{cfmcolor!0}\textbf{0}\\ \hline
\end{tabular}
>>> cm.getPDF('example.pdf')
The last command will produce a PDF example.pdf
, which will approzimately
look like the following:
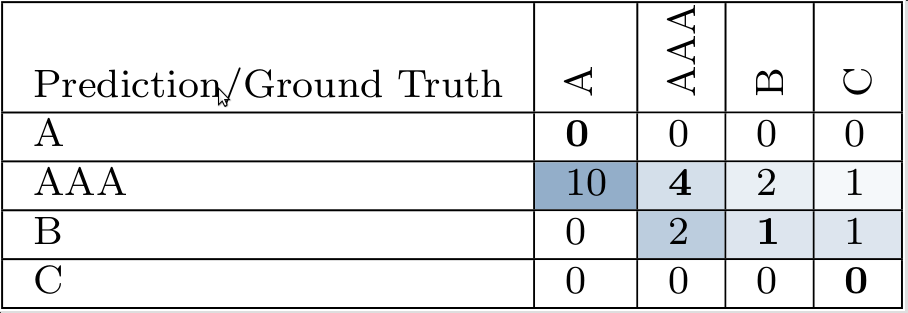